M. Geis, B. Sliwa, C. Bektas, C. Wietfeld, "TinyDRaGon: Lightweight Radio Channel Estimation for 6G Pervasive Intelligence", In 2022 IEEE Future Networks World Forum (FNWF), Montreal, Canada, October 2022.
- Best Paper Awards
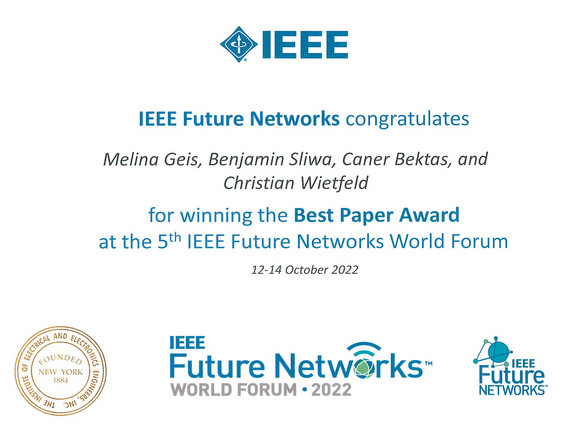
Due to the emerging challenges with future 6G networks such as high data rates and the need for remarkably low latency, future wireless communication systems must be planned extremely carefully and with respect to 6G’s various applications. Our previous work DRaGon has shown the potential of leveraging Machine Learning (ML)-based channel models, but its high model complexity lacks embedded system application. In this work, we present TinyDRaGon as a novel signal strength prediction method that combines expert knowledge from the mobile communications domain with lightweight ML methods to achieve accurate and computationally efficient predictions. In a comprehensive performance evaluation, the performance of TinyDRaGon is compared to both real-world measurements and a vast range of state-of-the-art channel modeling methods. It is found that TinyDRaGon achieves similar or even slightly better accuracy than its deep learning predecessor while also being ten times less time-consuming during training, less computationally expensive, and less energy-consuming. This makes TinyDRaGon a promising channel prediction candidate for pervasive intelligence within future 6G communication networks.