PhD on Machine-learning techniques for Car-to-Cloud Communications successfully completed
- News
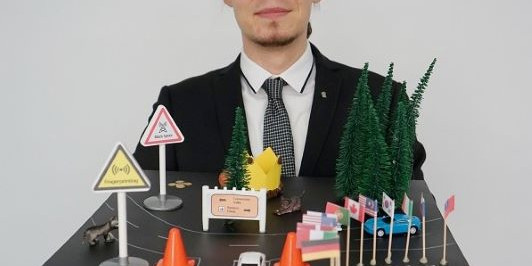
Vehicular big data is anticipated to become the “new oil” of the automotive industry. Although the novel vehicle-as-a-sensor paradigm will fuel the emergence of innovative crowdsensing-enabled services, the tremendously increased amount of transmitted data represents a massive challenge for the cellular network infrastructure and impacts the intra-cell coexistence of users and services that compete for the limited spectrum resources. More dramatically, due to the complex vehicular radio channel conditions, the mobile devices frequently need to reduce the transmission efficiency in favor of more reliable data transfer, ultimately resulting in a wastage of the limited network resources.
For the optimization and the performance evaluation of the novel transmission schemes developed in the scope of this thesis, data-driven network simulation is proposed as a new innovative methodology. Hereby, the combination of deterministic and probabilistic machine learning methods does not only result in a close to reality representation of concrete real-world evaluation scenarios, it also achieves a massive computational efficiency that is suitable for in-depth training of the reinforcement learning schemes.
As pointed out in a comprehensive real-world performance evaluation of the novel opportunistic data transfer methods, the apparently selfish goal of data rate maximization contributes to the good of all and allows to improve the intra-cell coexistence by significantly reducing the resource occupation time as well as the number of network resources per data packet. As a side-effect of the extensive utilization of highly reliable radio channel conditions, the communication-related energy consumption of the mobile device is also significantly improved. By adopting the model parameterization, the trade-off between the achievable benefits and the reduction of the data freshness inherently implied by the opportunistic medium access can be controlled with respect to the application requirements.